New publication
We have a new peer-reviewed publication in eLife in which we present retriever, a computational tool developed by Daniel that predicts candidate drugs and drug combinations by analyzing single-cell or bulk expression profiles from either a cohort of patients or based on individual samples.
- retriever leverages large drug perturbation datasets to find stable drug response signatures—ones that hold across treatment time, concentration, and cell types—corresponding to a disease of interest (see the figure below).
- We applied the method to triple-negative breast cancer (TNBC) data. First, we characterized differential expression between TNBC and healthy epithelial cells. These transcriptional changes form the basis for matching disease-specific signatures to retriever's drug response profiles.
- Next, we matched these TNBC-specific transcriptional changes to retriever's drug response signatures. This allowed us to rank candidate drugs that could counteract the disease-associated expression patterns. Two specific kinase inhibitors emerged as top ranked candidates.
- We tested this top predicted drug combination, as well as the individual drugs, in the lab and confirmed that this significantly reduced TNBC cell viability, supporting its ability to identify relevant candidates for drug repurposing.
- Importantly, retriever can be applied to data from individual patients. The top predicted drugs ranked highly in most patients, but other top-ranked drugs or combinations could offer insights into potential patient-specific treatment strategies.
- For detailed information, see the publications section. Also see the news article in eLife.
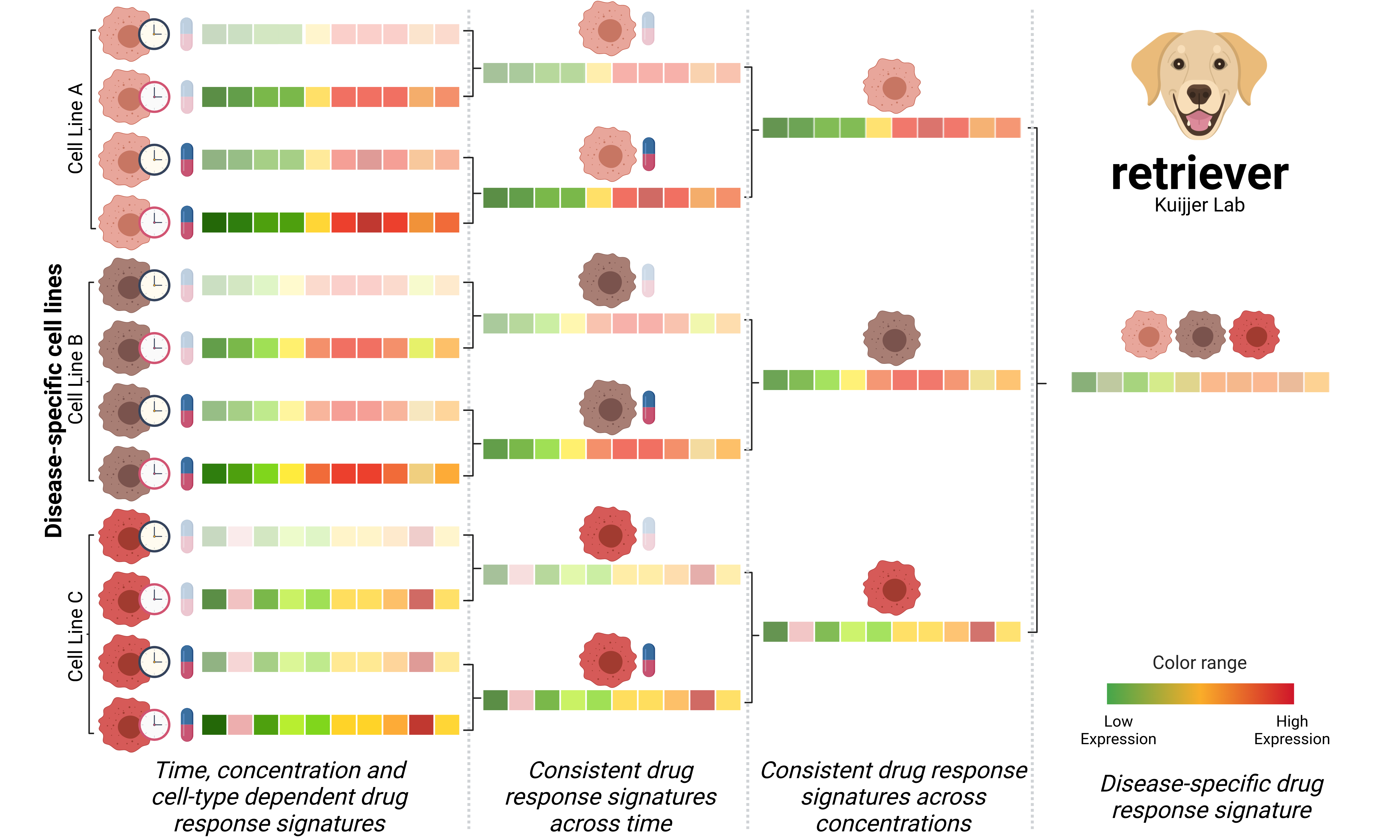