New publication
We have a new publication in Nature Computational Science, in which we present SCORPION, a new gene regulatory reconstruction algorithm for single-cell data. SCORPION's development was led by former group member led by Daniel.
- SCORPION's main asset is that it models genome-wide regulatory networks for both individual cell types and individual samples (see figure). This generates a large collection of networks that can then be used to, for example, statistically compare networks between groups of samples.
- We tested SCORPION in both synthetic and real-world scenarios. First, we benchmarked the coarse-grained data used in SCORPION using the BEELINE approach, and found it to outperform 12 other network inference approaches, both in accuracy and speed. Next, we evaluated SCORPION's accuracy by modeling networks for wild-type and transcription factor-perturbed cells. Here, we found that SCORPION accurately detects changes in transcription factor activity and their impact on target genes. We next tested SCORPION's scalability to population-level studies by modeling networks based on 200,436 cells from colorectal cancer & adjacent tissue. This detected regulatory interactions involved in cancer progression that aligned with our understanding of the disease. Finally, comparing left- with right-sided tumors, we found that targeting by the TF NFkB is associated with aggressiveness in right-sided tumors, which we could confirm in patient-derived xenograft models.
- The original publication can be found here. For more details, also refer to the publications section.
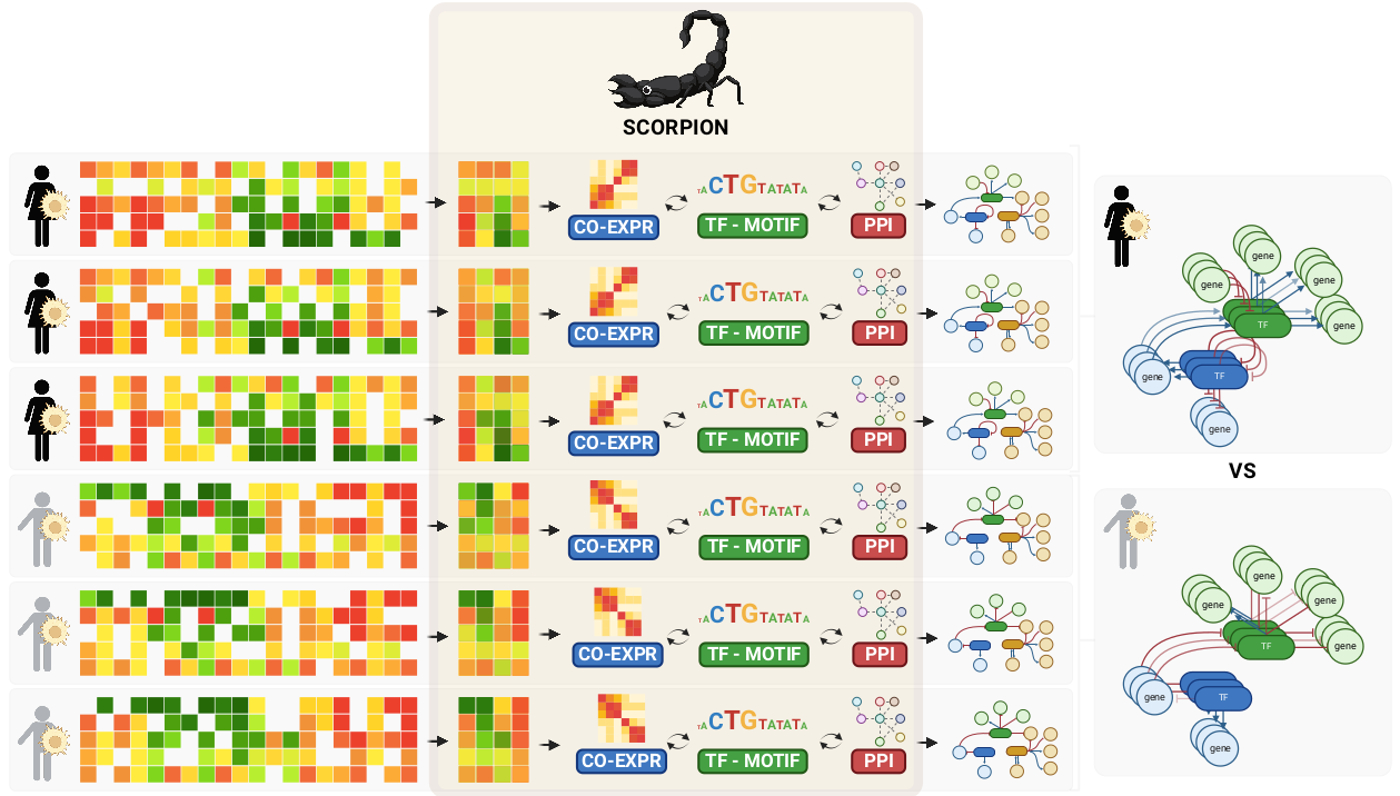